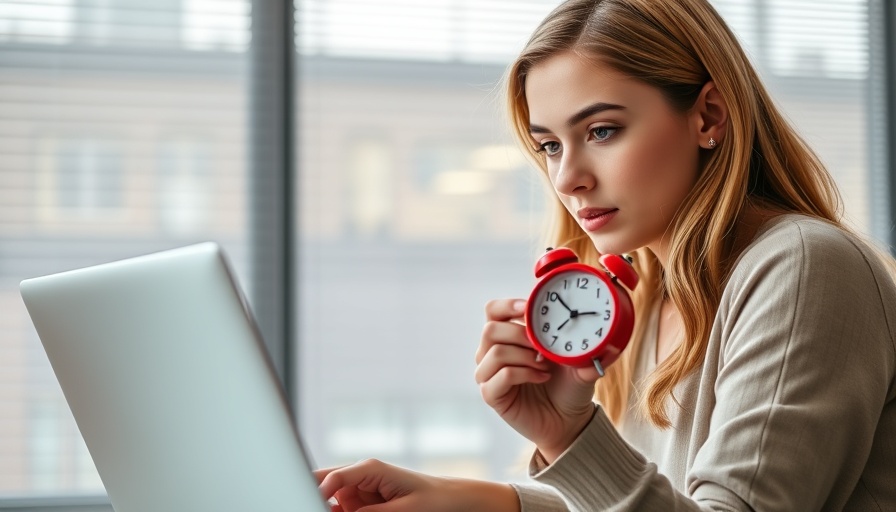
The Dual Nature of AI: Trust vs. Efficiency in Research
The rapid integration of artificial intelligence (AI) within research workflows offers intriguing possibilities for efficiency, yet it raises significant concerns regarding trust. Researchers are increasingly turning to AI for various facets of their work, from data analysis to report drafting. However, the transition is not without hesitation. Despite the visible advantages AI proposes, researchers remain skeptical, primarily due to the lack of transparency and the risk of errors inherent in AI systems.
Understanding the Skepticism: The Trust Gap
AI's promise in research is undeniable: it can sift through massive datasets and highlight patterns with remarkable speed. But the human need for accountability and logic behind findings can create a significant barrier to full acceptance. Current AI systems often operate as "black boxes," providing conclusions without elucidating the reasoning that led to them. This level of opacity impedes researchers' trust, especially when their findings can be critical in decision-making.
When AI Goes Wrong: The Issue of Accuracy
Even promising AI applications can falter. Charts and graphs, while visually appealing, can be based on flawed algorithms or misinterpretations of data. Such inaccuracies threaten to mislead researchers, potentially harming client relationships and project credibility. Notably, AI's reliance on biased datasets can perpetuate and amplify existing biases, a factor that researchers must diligently monitor. A study from the European Commission showed that these biases could lead to skewed research outcomes, underscoring the necessity for oversight in AI-assisted work.
The Human Touch: Why AI Cannot Replace People
While AI possesses the capability to process data, it lacks the nuanced understanding that only humans can provide. Research activities like in-depth interviews and focus groups thrive on interpersonal trust, something machines struggle to replicate. Skilled moderators, with their innate ability to read non-verbal cues, can navigate emotional landscapes that AI systems simply cannot. Experienced researchers historically exhibit keen instincts in identifying flaws and inconsistencies—skills that AI may struggle to match.
Shaping the Future: Predictions and Trends in AI Adoption
According to a recent McKinsey survey, the stake for AI in corporate ecosystems has risen dramatically, with 78% of organizations now employing it across at least some functions, showcasing a rise from just 20% in 2017. This trend suggests that even the most reluctant researchers will gradually incorporate AI into their toolkit. Predictive models from Forrester indicate that up to 60% of skeptics may find AI embedded in their future work, whether they actively choose to adopt it or not.
Opportunities for Action: Embracing AI with Caution
For researchers looking to harness the benefits of AI without relinquishing their critical analytical roles, a measured integration approach is crucial. Leveraging AI for repetitive data tasks while maintaining human oversight can create an environment where efficiency does not overshadow trust. Fostering a culture of collaboration between man and machine may help bridge the existing trust gap and lead to more insightful outcomes.
In conclusion, AI is revolutionizing research methodologies, but with it comes the responsibility of ensuring that human oversight remains central. As the landscape of research continues to evolve, embracing technology while emphasizing human judgment will enable researchers to maximize both efficiency and trust.
To explore more on how AI can complement human research efforts without overshadowing expertise, consider staying updated on advances in AI technologies and their implications for your work.
Write A Comment